WorldModelBench: The 1st Workshop on Benchmarking World Models
CVPR 2025 Workshop
Introduction
World models refer to predictive models of physical phenomena in the world surrounding us. These models are fundamental for Physical AI agents, enabling crucial capabilities such as decision-making, planning, and counterfactual analysis. Effective world models must integrate several key components, including perception, instruction following, controllability, physical plausibility, and future prediction. Over the past year, we have seen remarkable progress in building such world models – from video models trained with text-only conditioning to those leveraging richer conditioning sources (image, video, control). Research teams from both academia and industry have released numerous open-source and proprietary models.
This proliferation of world models opens doors to their use in several downstream applications, ranging from content creation, autonomous driving, to robotics. However, these models vary substantially in their training methodologies, data recipes, architectural designs, and input conditioning approaches. As a research community, we are compelled to critically examine their capabilities through comprehensive evaluation. This requires not only identifying relevant evaluation criteria (e.g., physical correctness, alignment with input prompts, generalizability) but also developing appropriate metrics and establishing standardized evaluation methodologies for fair assessment.
The goal of the WorldModelBench workshop is to provide a forum to facilitate in-depth discussions on evaluating world models. The workshop will cover a range of topics, including but not limited to:
- Designing accessible benchmarks for evaluating world models
- Designing methodology, protocols and metrics for quantitative evaluation
- Downstream evaluation of models through different tasks
- Considerations surrounding safety and bias in world models
Call For Papers
We welcome submissions on any aspects related to evaluating world-models, including but not limited to:
- Methods for developing world (and video) models, including novel architectures, training approaches, and scaling strategies
- Applications of world foundation models and video generation models to downstream embodied tasks, such as robotics and autonomous driving
- Novel metrics, benchmarks or datasets to evaluate world models
- Analysis of safety considerations and potential biases in world foundation models and video generation models
Submission Guideline:
- Submission website: openreview submission page
- Our workshop accepts both full paper submissions (4-8 pages excluding references) and extended abstract submissions (2-4 pages including references).
- Full paper submissions (4-8 pages excluding references) should NOT be published before. Please refer to CVPR 2025 author guidelines: https://cvpr.thecvf.com/Conferences/2025/AuthorGuidelines
- Submission Format: official CVPR template (double column; no more than 8 pages, excluding reference).
- Our paper reviewing process is double blind.
Important Dates (Anywhere on Earth)
Paper submission deadline | April 18th, 2025 |
Notifications to accepted papers | May 16th, 2025 |
Paper camera ready | May 23th, 2025 |
Schedule (Tentative)
Introduction and Opening Remarks | TBD |
Spotlight Presentation 1 | TBD |
Spotlight Presentation 2 | TBD |
Spotlight Presentation 3 | TBD |
Coffee Break | TBD |
Poster Session | TBD |
Invited Talk 1 | TBD |
Invited Talk 2 | TBD |
Invited Talk 3 | TBD |
Roundtable Discussion | TBD |
Invited Speakers
Wenhu Chen is a Professor at University of Waterloo and Vector Institute, also a Research Scientist at Google Deepmind. His research interest lies in natural language processing, deep learning and multimodal learning. He aims to design models to handle complex reasoning scenarios like math problem-solving, structure knowledge grounding, etc. He received the Area Chair Award in AACL-IJCNLP 2023, the Best Paper Honorable Mention in WACV 2021, and the UCSB CS Outstanding Dissertation Award in 2021.
Deepti Ghadiyaram is a Professor at Boston University and a member of Technical Staff at Runway. Her research focuses on improving the safety, interpretability, and robustness of AI systems. Previously she spent over 5 years at Meta AI Research working on image and video understanding models, fair and inclusive computer vision models, and ML explainability. She has served as a Program Chair for NeurIPS 2022 Dataset and Benchmarks track, hosted several tutorials and organized workshops and an Area Chair for CVPR, ICCV, ECCV, and NeurIPS.
Aditya Grover is a Professor at UCLA and a co-founder of Inception Labs. He leads the Machine Intelligence (MINT) group at UCLA to develop AI systems that can interact and reason with limited supervision. His current research is at the intersection of generative models and sequential decision making. He received many prestigious awards, such as NSF Career Award, Schmidt AI 2050 Early Career Fellowship, Kavli Fellow by the US National Academy of Sciences, Outstanding Paper Award at NeurIPS, etc.
Organizers
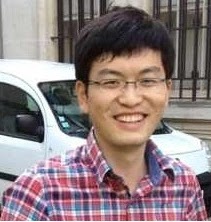
NVIDIA
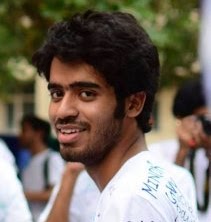
NVIDIA
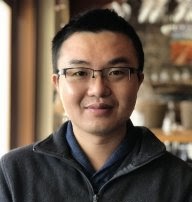
NVIDIA
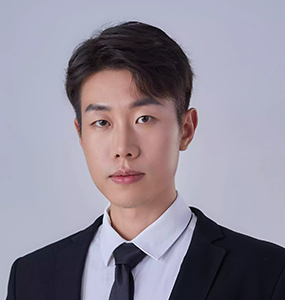
National University of Singapore
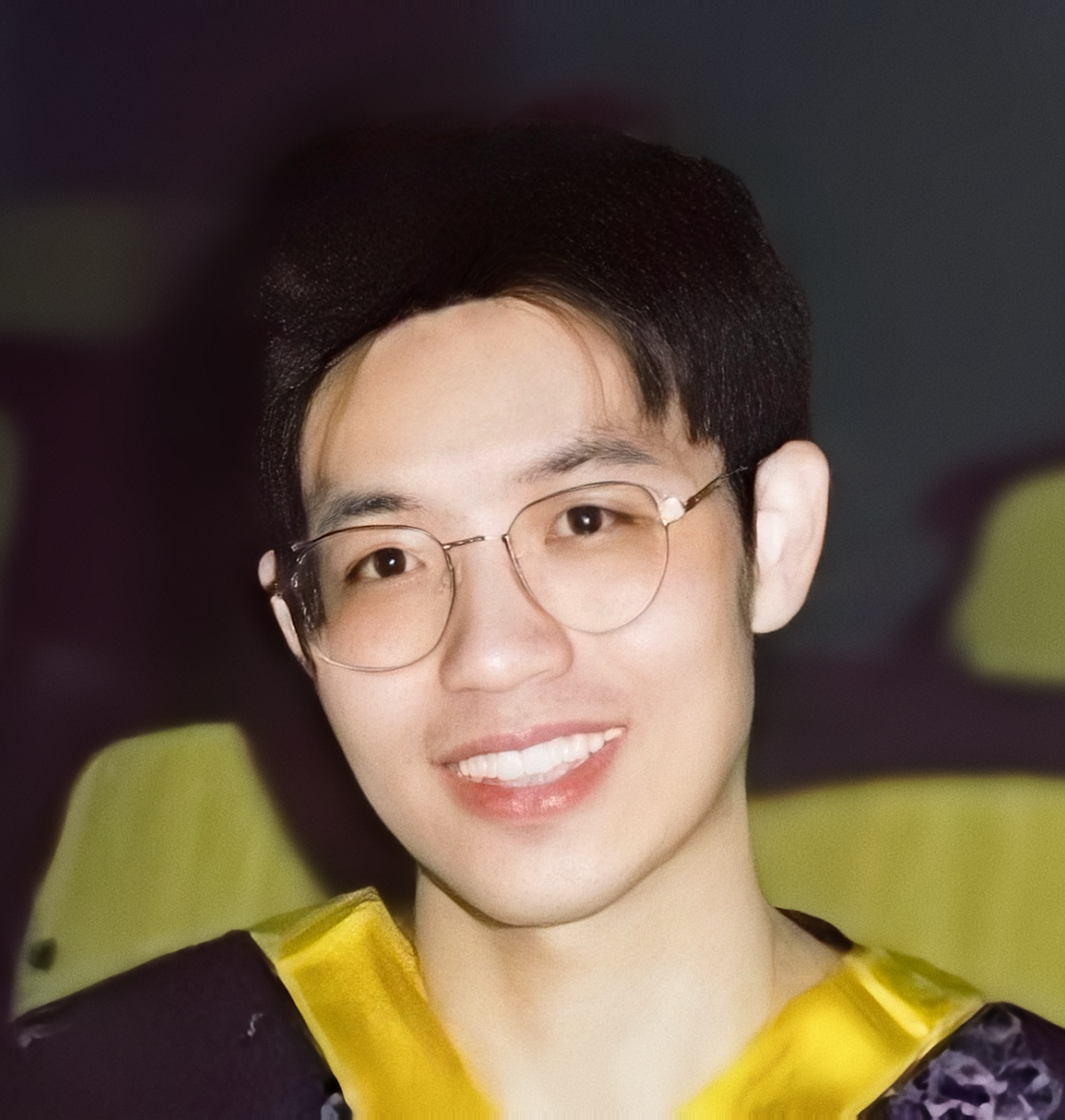
National University of Singapore
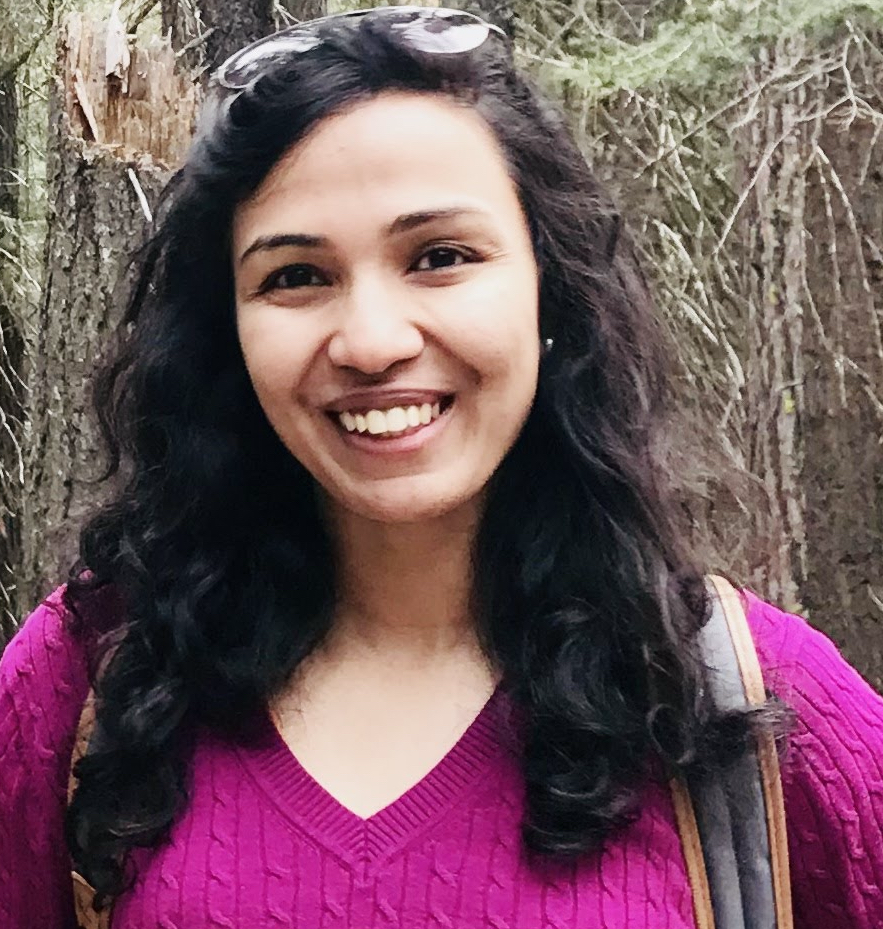
Boston University
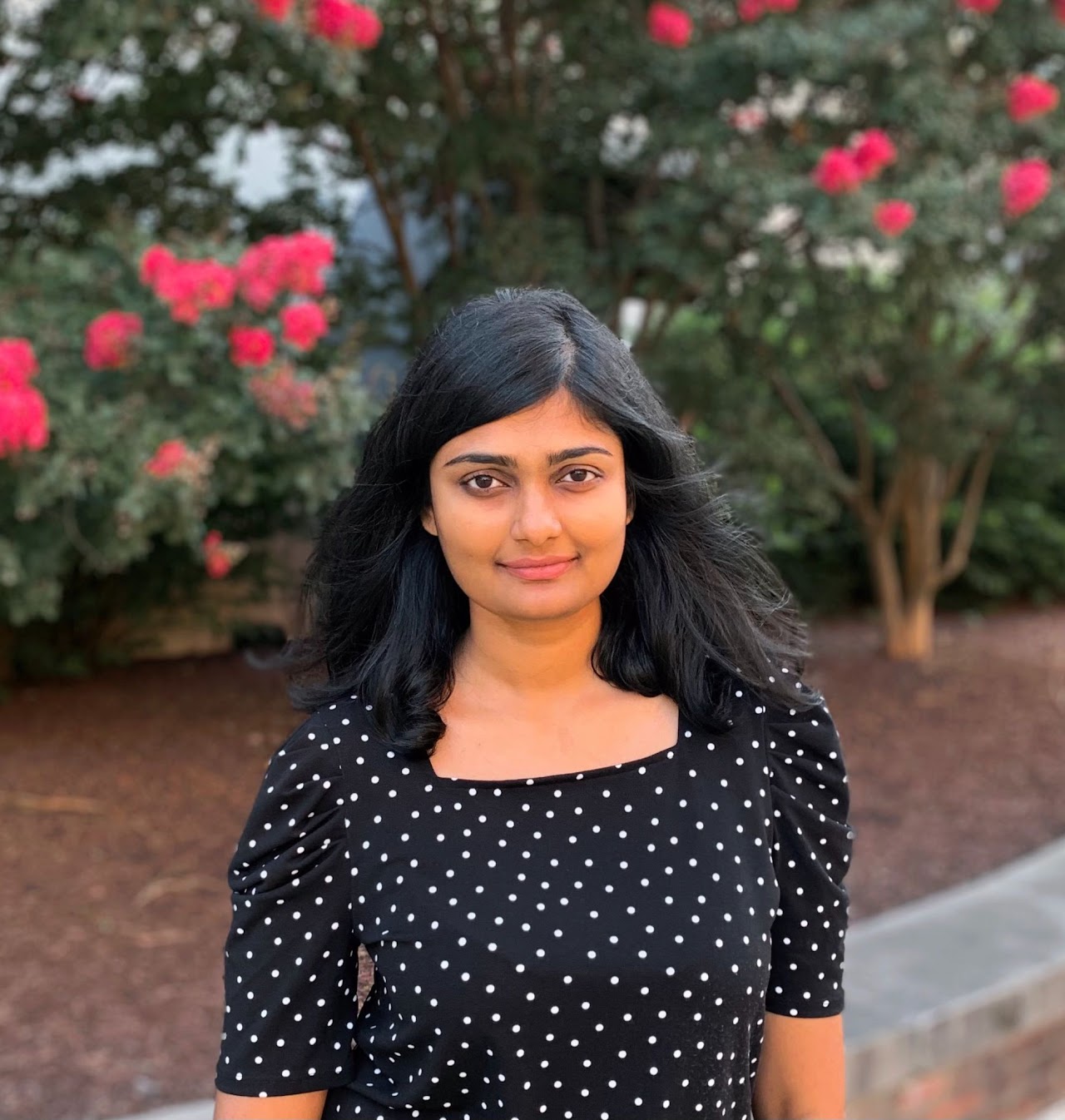
University of Maryland, College Park
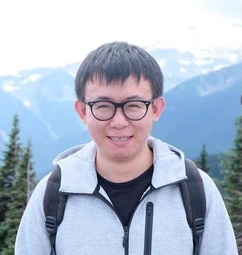
University of North Carolina at Chapel Hill
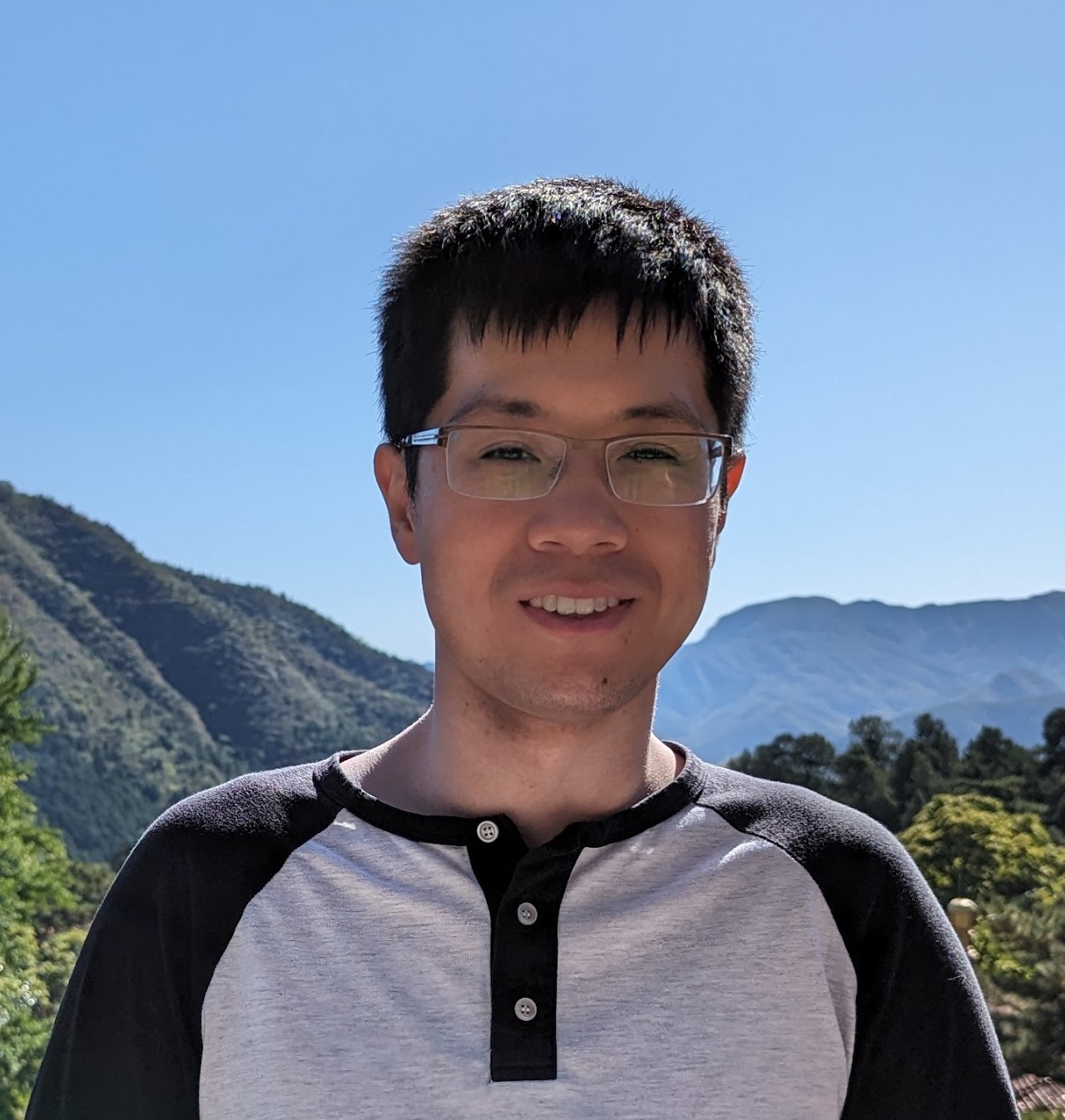
University of Waterloo
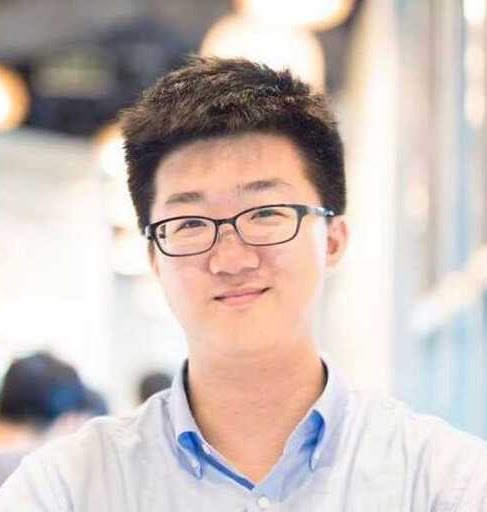
Luma AI
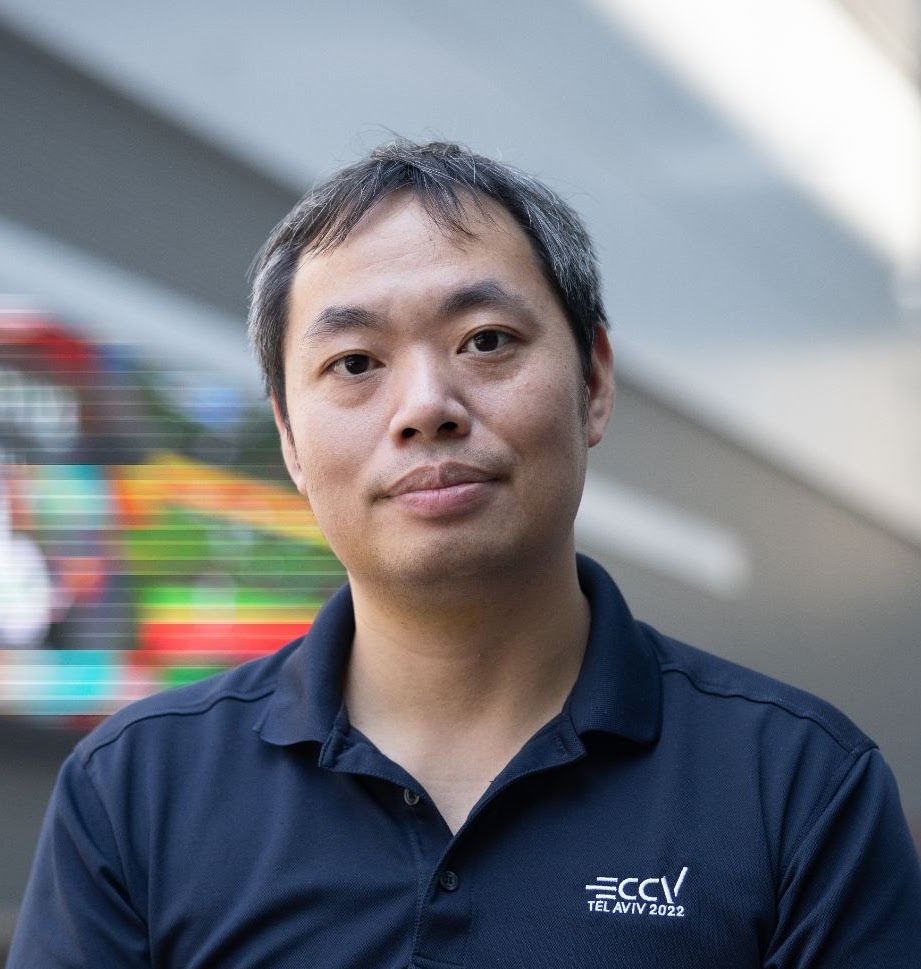
Georgia Tech
Contact
To contact the organizers please use worldmodelbench@gmail.com
Acknowledgments
Thanks to languagefor3dscenes for the webpage format.